Chapter 5 Hodgkin and Huxley Model
5.2 Introduction
Alan Hodgkin and Andrew Huxley were two of the most influential neuroscientists of the 20th century. The two met in 1938 at Trinity College, Cambridge where Huxley was an undergraduate student, and Hodgkin was a teacher. Huxley was only 21 years old, and Hodgkin was 24. Both of them were interested in understanding the particular dynamics of ion flow in neurons, so they began to conduct their own research as partners at the Plymouth Marine Laboratory in 1939.
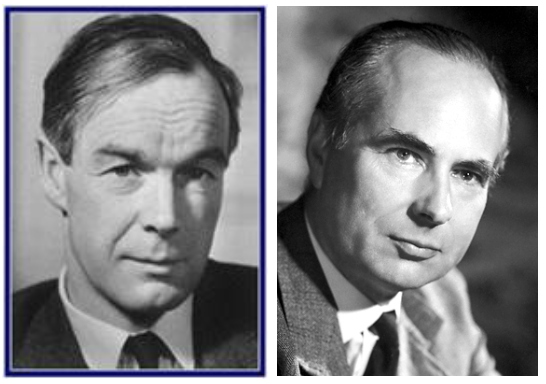
Figure 5.1: Alan Hodgkin (left) and Andrew Huxley (right).
Hodgkin and Huxley’s most important research centered around the activity of the giant axon of the longfin inshore squid. Squid are able to jet rapidly by contracting muscles in their mantle cavity, expelling water through a siphon on the underside of their body. This action is initiated by the giant axon. Since squid do not have a skull to constrain the growth of their neurons, this axon can measure up to 1.5 mm in diameter. Its large size means that its signal propagates extremely quickly, allowing the squid to rapidly respond to danger. The giant axon is hundreds of times larger than the largest axons in humans, making it much easier to study; it is visible to the naked eye and it is possible to insert the tip of a microelectrode into the membrane to record its activity. Hodgkin and Huxley took advantage of this fact to interrogate the electrochemical dynamics of neurons.
Hodgkin and Huxley used the voltage clamp technique to take their recordings. In this procedure, the giant axon is submerged in a solution of ions. Two wires are inserted into the membrane, and another is inserted into the solution. The two wires inside and outside the cell record the voltage, and the difference between these values represents the membrane potential. The third electrode is used to stimulate the neuron with varying amounts of current, and record the corresponding changes in voltage. Unfortunately, Hodgkin and Huxley took their first recordings in 1939 just weeks before Hitler invaded Poland, marking the start of World War II. Their research was postponed for seven years, but they were eventually able to continue their work and developed their beautiful set of equations (using only a hand-operated calculator) that attempt to describe the biological mechanisms of neuronal firing. Hodgkin and Huxley’s research greatly advanced our understanding of neuroscience, and they were awarded the Nobel Prize in Physiology of Medicine in 1963 for their work.
5.3 The Hodgkin and Huxley model
In simple terms, the Hodgkin-Huxley Model is an elaboration on the previously discussed Integrate and Fire Model. While the Integrate and Fire Model only accounts for a single “leak” current when modeling action potentials, the Hodgkin-Huxley Model acknowledges the role of ionic sodium and potassium currents in addition to leak currents through the use of conductance terms, known as gating variables.

Figure 5.2: Idealized voltage-gated potassium (left) and sodium (right) channels. Each channel has four independent subunits that are comprised of different gating variables (n, m, or h).
For the purposes of this model, voltage-gated potassium and sodium channels consist of four independent subunits, and each of these subunits are comprised of a gating variable, n, m, or h, as seen in Figure 5.2. The value of these gating variables represents the probability that an ion channel is open at a given voltage.
Voltage-gated potassium channels are modeled by the gating variable for potassium activation, n. Because potassium channels have a single gating variable that makes up each of the four subunits, n is raised to the fourth power. The conductance of voltage-gated potassium channels is modeled below: \[\bar{g}_{K}n^{4}(V(t)-E_{K}) \]
Voltage-gated sodium channel activation is modeled by the gating variables for sodium activation and inactivation, which are represented as m and h, respectively. m makes up three of the independent subunits and, as a result, is raised to the third power. On the other hand, h, which occurs when the action potential approaches its peak, makes up a single subunit. The combination of m and h gives rise to the conductance of the voltage-gated sodium channel, modeled below: \[\bar{g}_{Na}m^{3}h(V(t)-E_{Na}) \]
The final conductance taken into account by Hodgkin and Huxley is the leak potential of all the ions when all ion channels are open. This conductance of the leak channels is represented below: \[\bar{g}_{L}(V(t)-E_{L}) \]
These three conductance variables are combined together to form the Hodgkin-Huxley equation which is written as follows: \[C\frac{dV}{dt}=I_{e}(t)-[(\bar{g}_{Na}m^{3}h(V(t)-E_{Na}))+(\bar{g}_{K}n^{4}(V(t)-E_{K}))+(\bar{g}_{L}(V(t)-E_{L}))] \]
Expression | Meaning |
---|---|
n | Potassium gating variable |
m | Sodium activation gating variable |
h | Sodium inactivation gating variable |
\(C_m\) | Specific membrane capacitance |
\(I_e\) | Injected current |
\(\bar{g}_{Na}\) | Maximum Na+ conductance |
\(\bar{g}_{K}\) | Maximum K+ conductance |
\(\bar{g}_{L}\) | Maximum leak conductance |
\(V_m\) | Membrane potential |
\(E_{Na}\) | Sodium Nernst potential |
\(E_{K}\) | Potassium Nernst potential |
\(E_{L}\) | Leak Nernst potential |

Figure 5.3: Each of an ion channel’s subunits can either be in a permissive or non-permissive state. Movement from a permissive state to a non-permissive state is denoted as \(\beta\), whereas movement from a non-permissive state to a permissive state is denoted as \(\alpha\).
Each of the aforementioned subunits of voltage-gated potassium and sodium channels can be in one of two states: permissive or non-permissive. If a subunit is in a permissive state, the probability that the ion channel is open is increased, with the opposite being true for a subunit in a non-permissive state. The rate of movement from a permissive state to a non-permissive state is expressed as rate constant \(\beta\), and the movement from a non-permissive to permissive state is expressed as rate constant \(\alpha\). Below are the equations for both rate constants for each gating variable:
\[ \alpha_{n}(V_{m}) = \frac{0.01(V_{m}+55)}{1-exp(-0.1(V_{m}+55))} \] \[\alpha_{m}(V_{m}) = \frac{0.1(V_{m}+40)}{1-exp(-0.1(V_{m}+40))}\] \[\alpha_{h}(V_{m}) = 0.07exp(-0.05(V_{m}+65)) \] \[\beta_{n}(V_m) = 0.125exp(-0.0125(V_{m}+65)) \] \[\beta_{m}(V_{m}) = 4exp(-0.0556(V_{m}+65)) \] \[\beta_{h}(V_{m}) = \frac{1}{1+exp(-0.1(V_{m}+35))} \]
Expression | Meaning |
---|---|
\(\alpha_n\) | Rate constant for K+ channel opening |
\(\alpha_m\) | Rate constant for Na+ activation gate opening |
\(\alpha_h\) | Rate constant for Na+ inactivation gate opening |
\(\beta_n\) | Rate constant for K+ channel closing |
\(\beta_m\) | Rate constant for Na+ activation gate closing |
\(\beta_h\) | Rate constant for Na+ inactivation gate closing |
Given this information, we can calculate the value of each gating variable over different voltages and times: \[m\frac{dV}{dt} = \alpha_{m}(V)(1-m)-\beta_{m}(V)m \]
Both n and h can be substituted for m in the above equation in order to calculate values for each gating variable.
Worked Example:
Have you ever wondered how anesthesia makes a tooth extraction painless? It’s because anesthesia works by blocking the activation of voltage-dependent Na+ channels. This prevents the propagation of the action potentials that carry that awful pain sensation.
Using the equations below, calculate the maximum conductances of each ion in the resting state.
Useful parameters:
- \(V_m\) = -68 mV
- \(C_m\) = -20.1 nF
- \(E_L\) = -54 mV
- \(E_{Na}\) = 50 mV
- \(E_K\) = -77 mV
- \(R_L = 1/3 M\Omega\)
- \(g_{Na} = 1200 mS/mm^2\)
Equations:
\[I_{ion} = g_{Na} \cdot m^3h(V_m-E_{Na}) + g_K \cdot n^4 (V_m-E_K) + g_L \cdot (V_m-E_L)\]
\[\frac{dn}{dt} = \alpha_n(V) \cdot (1-n) - \beta_n(V) \cdot(n)\]
\[\frac{dm}{dt} = \alpha_m(V) \cdot (1-m) - \beta_m(V) \cdot(m)\]
\[\frac{dh}{dt} = \alpha_h(V) \cdot (1-h) - \beta_h(V) \cdot(h)\]
Step 1: Determine necessary equations The resting potential can be considered to be a steady state because the voltage is not changing. Therefore, \(\frac{dp}{dt}=0\) for p={n, m, h} and therefore the last three equations will not be used.
Step 2: Calculate n, m, and h We need to now use the resting potential to solve for the steady state values of the gating variables.
\[p_\infty = \frac{\alpha_p}{\alpha_p+\beta_p}\]
for p = {n, m, h}
Therefore, we need to calculate each \(\alpha\) and \(\beta\).
\[ \alpha_{n}(V_{m}) = \frac{0.01(V_{m}+55)}{1-exp(-0.1(V_{m}+55))} \] \[\alpha_{n}(V_{rest}) = \frac{0.01(-68+55)}{1-exp(-0.1(-68+55))} \] \[\alpha_{n} = 0.049\]
\[\alpha_{m}(V_{m}) = \frac{0.1(V_{m}+40)}{1-exp(-0.1(V_{m}+40))}\] \[\alpha_{m}(V_{rest}) = \frac{0.1(-68+40)}{1-exp(-0.1(-68+40))}\] \[\alpha_{m}(V_{rest}) = 0.18\]
\[\alpha_{h}(V_{m}) = 0.07exp(-0.05(V_{m}+65)) \] \[\alpha_{h}(V_{rest}) = 0.07 \cdot exp(-0.05 \cdot(-68+65)) \] \[\alpha_{h}(V_{rest}) = 0.08\]
\[\beta_{n}(V_m) = 0.125exp(-0.0125(V_{m}+65)) \] \[\beta_{n}(V_{rest}) = 0.125 \cdot exp(-0.0125 \cdot(-68+65)) \] \[\beta_{n}(V_{rest}) = 0.13\]
\[\beta_{m}(V_{m}) = 4exp(-0.0556(V_{m}+65)) \] \[\beta_{m}(V_{rest}) = 4 \cdot exp(-0.0556 \cdot(-68+65)) \] \[\beta_{m}(V_{rest}) = 4.73\]
\[\beta_{h}(V_{m}) = \frac{1}{1+exp(-0.1(V_{m}+35))} \] \[\beta_{h}(V_{rest}) = \frac{1}{1+exp(-0.1(-68+35))} \] \[\beta_{h}(V_{rest}) = 0.036\]
Now, with each of our \(\alpha\) and \(\beta\) values, we can calculate our gating variables:
\[n_\infty = \frac{\alpha_n}{\alpha_n+\beta_n}\] \[n_\infty = \frac{0.049}{0.049+0.13} = 0.274\]
\[m_\infty = \frac{\alpha_m}{\alpha_m+\beta_m}\] \[m_\infty = \frac{0.18}{0.18+4.73} = 0.037\]
\[h_\infty = \frac{\alpha_h}{\alpha_h+\beta_h}\] \[h_\infty = \frac{0.08}{0.08+0.036} = 0.690\]
Step 3: Calculate \(g_L\) from resistance units. Remember that \(g = \frac{1}{R}\)! \[g_L = (1/R) = (1/(1/3)) = 3 mS/mm^2\] Step 4: Solve for \(g_K\) Kirchhoff’s Law states that the algebraic sum of all the currents entering and leaving a junction must be equal to 0. Therefore:
\[0 = g_{Na} \cdot m^3h(V_m-E_{Na}) + g_K \cdot n^4 (V_m-E_K) + g_L \cdot (V_m-E_L)\]
And we may plug in the values that we already have:
\[0 = 1200 \cdot 0.037^30.69 \cdot(-68-50) + g_K \cdot 0.274^4 \cdot (-68--77) + 3 \cdot (-68--54.387)\]
\[0 = 4.956 + g_K \cdot 0.051 + (-40.84)\]
\[g_K = 703.6\]
Worked Example: The voltage of a neuron is clamped at -20 mV, depolarized from its resting potential of -65 mV. The steady-state values of the gating variables in the two conditions are shown below. Comment on what these changes mean for the neuron’s behavior.
V=-65 mV | V=-20mV |
---|---|
m=0.0529 | m=0.875 |
n=0.3177 | n=0.820 |
h=0.5961 | h=0.009 |
Answer The value of m represents the probability of voltage-gated Na+ channels to be open, which increases as the cell depolarizes. The n value represents the probability that the voltage-gated K+ channel is open and also increases with depolarization, but not to the same extent because K+ channels are slower to open and close. The h values represent the probability of Na+ channel inactivation, which decreases significantly with depolarization because we have not hit the peak of the action potential. As a result, it is fair to assume that the h value will increase as we approach the peak of the action potential.
5.4 Summary
In this chapter, we discuss Hodgkin and Huxley’s journey to discovery, the intricacies of their method, and will later examine some modern applications of their model through code. Using the voltage-clamp technique and squid axons as their vehicle of study, the two scientists were able to provide a model for the biological mechanisms of neuronal firing in an action potential. Additionally, by implementing voltage-gated sodium and potassium pumps, Hodgkin and Huxley were able to create a model that was much more accurate than the leaky integrate and fire model discussed in the previous chapter. Hodgkin and Huxley’s work continues to serve as a fundamental model of computational neuroscience, but still fails to account for the irregular firing rate of an actual neuron.
5.5 Exercises:
5.5.1 Conceptual Exercises:
- Describe the primary difference between the leaky integrate and fire model and the Hodgkin and Huxley model.
- What do the gating variables n, m, and h represent? What does it mean when a gating variable has a higher or lower value?
- What do the rate constants \(\alpha\) and \(\beta\) represent?
- Determine which of the variables in the Hodgkin and Huxley model are constants, given, or need to be solved for. Then, determine the order in which to solve for the unknown variables.
5.5.2 Coding Exercises:
Welcome to the coding problems for the Hodgkin and Huxley model! These exercises will help you get comfortable with using the Hodgkin and Huxley Model in different settings. To get started, simply run the following cell to create a few custom functions that we will be using for these exercises. A brief description of the functions can be found below:
- HHmodel: Calculates and plots the Hodgkin-Huxley model with parameters that we will pass through the function. These parameters include the injected current (Ie), time steps (dt), the final time interval (tFinal), the time at which the stimulus starts (tStimStart), the time as which the stimulus ends (tStimEnd), and the resting potential of the neuron (RestingPotential).
- HHmodel_threshold: Determines whether or not an injected current that we pass through the function reaches the required threshold potential to fire. The only parameter of this function is the injected current (Ie).
- HHmodel_compare: Compares differences in neuronal activity when injected with different currents. The parameters of this function include the injected currents (Ie) and the resting potential of the neuron (RestingPotential).
No changes to the code are needed, and there is no expected output at this time, but feel free to look through the code and descriptive comments to see how each aspect of the model is implemented!
# Import necessary libraries
import numpy as np
import matplotlib.pyplot as plt
#Creates a custom Hodgkin and Huxley function
def HHmodel(Ie, dt, tFinal, tStimStart, tStimEnd, RestingPotential):
import matplotlib.pyplot as plt
# Defines the model parameters
= 10 # capacitance per unit area (nF/mm^2)
c = 3 # leak maximal conductance per unit area (mS/mm^2)
gMaxL = -54.387 # leak conductance reversal potential (mV)
EL = 360 # maximal K conductance per unit area (mS/mm^2)
gMaxK = -77 # K conductance reversal potential (mV)
EK = 1200 # maximal Na conductance per unit area (mS/mm^2)
gMaxNa= 50 # Na conductance reversal potential (mV)
ENa
# sets up data structures to hold relevant variable vectors
= np.arange(0,tFinal, dt)
timeVec = np.zeros(len(timeVec))
voltageVector = np.zeros(len(timeVec))
Ivector = np.zeros(len(timeVec))
mVec = np.zeros(len(timeVec))
hVec = np.zeros(len(timeVec))
nVec = np.zeros(len(timeVec))
tauVec
# assigns the initial value of each variable
0] = RestingPotential
voltageVector[0] = 0.0529
mVec[0] = 0.5961
hVec[0] = 0.3177
nVec[
# defines the stimulus
= int(tStimStart/dt)
tStimStart = int(tStimEnd/dt)
tStimEnd = Ie
Ivector[tStimStart:tStimEnd]
# For-loop integrates equations into model
for v in range(len(timeVec)-1):
# We include -1 because we already have the first value
# Calculates alpha values for m, h, and n
= 0.01*(voltageVector[v] + 55) /
alpha_n 1-np.exp(-0.1*(voltageVector[v]+55)))
(= (0.1*(voltageVector[v]+40))/
alpha_m 1-np.exp(-0.1*(voltageVector[v]+40)))
(= 0.07*np.exp(-.05*(voltageVector[v]+65))
alpha_h
# Calculates beta values for m, h, and n
= 0.125*np.exp(-.01125*(voltageVector[v]+55))
beta_n = 4*np.exp(-.05556*(voltageVector[v]+65))
beta_m = 1 / (1 + np.exp(-0.1*(voltageVector[v]+35)))
beta_h
# Calculates tau (time constant) values for m, h, and n
= 1 / (alpha_n + beta_n)
tau_n = 1 / (alpha_m + beta_m)
tau_m = 1 / (alpha_h + beta_h)
tau_h
# Calculates infinity values for m, h, and n
= alpha_m/(alpha_m + beta_m)
pm = alpha_n/(alpha_n+ beta_n)
pn = alpha_h/(alpha_h + beta_h)
ph
# Calculates and store values in m, h, and n vectors
+1] = pm + (mVec[v] - pm)*np.exp(-dt/tau_m)
mVec[v+1] = pn + (nVec[v] - pn)*np.exp(-dt/tau_n)
nVec[v+1] = ph + (hVec[v] - ph)*np.exp(-dt/tau_h)
hVec[v
# Updates Vinf and tauV
= gMaxL + gMaxK*(nVec[v]**4) +
denominator *(mVec[v]**3)*hVec[v]
gMaxNa= c / denominator
tauV = ((gMaxL)*EL + gMaxK*(nVec[v]**4)*EK + \
Vinf *(mVec[v]**3)*hVec[v]*ENa + Ivector[v])/denominator
gMaxNa
# Calculates and store next voltage value in vector
+1] = Vinf + (voltageVector[v]-Vinf)*np.exp(-dt/tauV)
voltageVector[v
# Plotting
1, figsize=(10,10))
plt.figure(4,1,1)
plt.subplot(
plt.plot(timeVec,voltageVector)'Hodgkin and Huxley Simulation')
plt.title('Voltage in mV')
plt.ylabel(4,1,2)
plt.subplot(
plt.plot(timeVec, mVec)'g_Na activation variable m')
plt.ylabel(4,1,3)
plt.subplot(
plt.plot(timeVec, hVec)'g_Na inactivation variable h')
plt.ylabel(4,1,4)
plt.subplot(
plt.plot(timeVec, nVec)'g_K activation variable')
plt.ylabel('Time in ms') plt.xlabel(
#creates a custom function that will determine the minimum Ie
# required to surpass the threshold potential
def HHmodel_threshold(Ie):
= 250
tStimStart = 750
tStimEnd = 0.1 # time step (ms)
dt = 1000 # total time of run (ms)
tFinal = -65
RestingPotential = 10 # capacitance per unit area (nF/mm^2)
c = 3 # leak maximal conductance per unit area (mS/mm^2)
gMaxL = -54.387 # leak conductance reversal potential (mV)
EL = 360 # maximal K conductance per unit area (mS/mm^2)
gMaxK = -77 # K conductance reversal potential (mV)
EK = 1200 # maximal Na conductance per unit area (mS/mm^2)
gMaxNa= 50 # Na conductance reversal potential (mV)
ENa
for current in range(len(Ie)):
= np.arange(0,tFinal, dt)
timeVec = np.zeros(len(timeVec))
voltageVector = np.zeros(len(timeVec))
Ivector = np.zeros(len(timeVec))
mVec = np.zeros(len(timeVec))
hVec = np.zeros(len(timeVec))
nVec = np.zeros(len(timeVec))
tauVec 0] = RestingPotential
voltageVector[0] = 0.0529
mVec[0] = 0.5961
hVec[0] = 0.3177
nVec[
2499:7499] = Ie[current]
Ivector[
for v in range(len(timeVec)-1):
= 0.01*(voltageVector[v] + 55)/ \
alpha_n 1-np.exp(-0.1*(voltageVector[v]+55)))
(= (0.1*(voltageVector[v]+40))/ \
alpha_m 1-np.exp(-0.1*(voltageVector[v]+40)))
(= 0.07*np.exp(-.05*(voltageVector[v]+65))
alpha_h = 0.125*np.exp(-.01125*(voltageVector[v]+55))
beta_n = 4*np.exp(-.05556*(voltageVector[v]+65))
beta_m = 1 / (1 + np.exp(-0.1*(voltageVector[v]+35)))
beta_h = 1 / (alpha_n + beta_n)
tau_n = 1 / (alpha_m + beta_m)
tau_m = 1 / (alpha_h + beta_h)
tau_h = alpha_m/(alpha_m + beta_m)
pm = alpha_n/(alpha_n+ beta_n)
pn = alpha_h/(alpha_h + beta_h)
ph +1] = pm + (mVec[v] - pm)*np.exp(-dt/tau_m)
mVec[v+1] = pn + (nVec[v] - pn)*np.exp(-dt/tau_n)
nVec[v+1] = ph + (hVec[v] - ph)*np.exp(-dt/tau_h)
hVec[v= gMaxL + gMaxK*(nVec[v]**4) +
denominator *(mVec[v]**3)*hVec[v]
gMaxNa= c / denominator
tauV = ((gMaxL)*EL + gMaxK*(nVec[v]**4)*EK + \
Vinf *(mVec[v]**3)*hVec[v]*ENa + Ivector[v])/ denominator
gMaxNa+1] = Vinf + \
voltageVector[v-Vinf)*np.exp(-dt/tauV)
(voltageVector[v]
# Checks to see if the given current resulted in an
# action potential
# Values around 25 mv is only reached if the neuron spikes.
# A value of 20 would similarly be appropriate
if voltageVector[v] > 25:
return(print("With an external current of", Ie[current]-1 ,
"nA/mm^2 threshold potential was finally reached!"))
return(print("Looks like you didn't provide a large value in your range
caused the neuron to spike. Try again!"))
# plots the behavior of a neuron when injected with different
# currents on the same graphs
def HHmodel_compare(Ie, RestingPotential):
= 0
terminate = 10
tStimStart = 30
tStimEnd = 50
tFinal = 0.002
dt = 10
c = 0.003e03
gMaxL = -54.387
EL = 0.36e03
gMaxK = -77
EK = 1.2e03
gMaxNa = 50
ENa
for current in range(len(Ie)):
= np.arange(0,tFinal, dt)
timeVec = np.zeros(len(timeVec))
voltageVector = np.zeros(len(timeVec))
Ivector = np.zeros(len(timeVec))
mVec = np.zeros(len(timeVec))
hVec = np.zeros(len(timeVec))
nVec = np.zeros(len(timeVec))
tauVec
0] = RestingPotential
voltageVector[
0] = 0.0529
mVec[0] = 0.5961
hVec[0] = 0.3177
nVec[
5000:15000] = Ie[current]
Ivector[
for v in range(len(timeVec)-1):
= 0.01*(voltageVector[v] + 55) / \
alpha_n 1-np.exp(-0.1*(voltageVector[v]+55)))
(= (0.1*(voltageVector[v]+40))/ \
alpha_m 1-np.exp(-0.1*(voltageVector[v]+40)))
(= 0.07*np.exp(-.05*(voltageVector[v]+65))
alpha_h = 0.125*np.exp(-.01125*(voltageVector[v]+55))
beta_n = 4*np.exp(-.05556*(voltageVector[v]+65))
beta_m = 1 / (1 + np.exp(-0.1*(voltageVector[v]+35)))
beta_h = 1 / (alpha_n + beta_n)
tau_n = 1 / (alpha_m + beta_m)
tau_m = 1 / (alpha_h + beta_h)
tau_h = alpha_m/(alpha_m + beta_m)
pm = alpha_n/(alpha_n+ beta_n)
pn = alpha_h/(alpha_h + beta_h)
ph +1] = pm + (mVec[v] - pm)*np.exp(-dt/tau_m)
mVec[v+1] = pn + (nVec[v] - pn)*np.exp(-dt/tau_n)
nVec[v+1] = ph + (hVec[v] - ph)*np.exp(-dt/tau_h)
hVec[v= gMaxL + gMaxK*(nVec[v]**4) + \
denominator *(mVec[v]**3)*hVec[v]
gMaxNa= c / denominator
tauV = ((gMaxL)*EL + gMaxK*(nVec[v]**4)*EK + \
Vinf *(mVec[v]**3)*hVec[v]*ENa + Ivector[v])/
gMaxNa
denominator +1] = Vinf +
voltageVector[v-Vinf)*np.exp(-dt/tauV)
(voltageVector[v]
# stores values so the two plots can be superimposed
if terminate ==0:
= mVec
Mv = nVec
Nv = hVec
Hv = voltageVector
Vv = 1
terminate
#plotting
1, figsize=(10,10))
plt.figure(4,1,1)
plt.subplot(
plt.plot(timeVec,voltageVector, timeVec, Vv)'Hodgkin and Huxley Simulation')
plt.title('Voltage in mV')
plt.ylabel(4,1,2)
plt.subplot(
plt.plot(timeVec, mVec, timeVec, Mv)'g_Na activation variable m')
plt.ylabel(4,1,3)
plt.subplot(
plt.plot(timeVec, hVec, timeVec, Hv)'g_Na inactivation variable h')
plt.ylabel(4,1,4)
plt.subplot(
plt.plot(timeVec, nVec, timeVec, Nv)'g_K activation variable')
plt.ylabel('Time in ms')
plt.xlabel(
print("Cell run successfully! Please proceed to the next part")
Problem 1: Exploring the Model Part 1 What can the model do? The function HHmodel() provides insight by simulating a neuron firing and the behavior of its channel gating variables under various conditions. Simulate an experiment that runs for 500 ms in which a neuron is exposed to a current of 100 \(nA/mm^2\) from 100-400 ms. Use time steps of 0.1 ms and set the resting potential to -65 mV.
Note, the code has been started for you - set the variables to their specified values and run the code.
= # time to start injecting current (ms)
tStimStart = # time to end injecting current (ms)
tStimEnd = # total time of run (ms)
tFinal = # time step (ms)
dt = # nA/mm^2
Ie = #mv
RestingPotential
# Run the custom function
HHmodel(Ie, dt, tFinal, tStimStart, tStimEnd, RestingPotential)
Part 2 Nice work! Does increasing the injected current increasing the firing rate? Using the same code, increase the injected current to 250 \(nA/mm^2\) to find out!
Part 3, optional Play around with the parameters to see how changing the neuron’s starting conditions will affect its behavior!
Problem 2: Threshold Potential Part 1 Neurons operate on a “all or nothing” basis, meaning they fire an action potential at full force or do not fire at all. The function HHmodel_threshold() takes different values of injected currents (\(I_e\)) and returns the minimum value that elicited an action potential. Using np.arange(), set \(I_e\) equal to a vector of increasing voltages to determine the minimum injected current required for the neuron to cross its threshold potential. Hint: first define your external stimulation, \(I_e\) then use the HHmodel_threshold() function.
Part 2 The function HHmodel_compare() will plot the behavior of a neuron and its gating variables for two different injected current values. The function’s input is the array \(I_e\) that contains two values. Using the external current determined in part one and the preceding integer, run the code to see the difference between just missing and passing the threshold potential!
Part 3 Pretty striking difference right? Now imagine there is a neuron where the resting potential is -60 mV rather than -65. What would happen if all else remained equal? Change the resting potential to -60 mV and rerun the code to find out!(Note: HHmodel_compare() also accepts an input for the resting potential.)
Problem 3: Pick your Poison(s) Part 1 The poisonous dart frog of Central and South America secretes the neurotoxin batrachotoxin (BTX). Exposure to BTX results causes paralysis by irreversibly binding to the sodium channels and preventing them from closing. In the following code, simulate a neuron’s exposure to BTX by setting the relevant variable to the appropriate value. Note: the only code that should be altered has been sectioned off using hashtags.
# Define input parameters
= 10
c = 0.003e03
gMaxL = -54.387
EL = 0.36e03
gMaxK = -77
EK = 1.2e03
gMaxNa = 50
ENa = 200
Ie = 1000
tFinal
# Set up data structures
= np.arange(0,tFinal, dt)
timeVec = np.zeros(len(timeVec))
voltageVector = np.zeros(len(timeVec))
Ivector = np.zeros(len(timeVec))
mVec = np.zeros(len(timeVec))
hVec = np.zeros(len(timeVec))
nVec = np.zeros(len(timeVec))
tauVec
# Initialize starting values
0] = -65
voltageVector[0] = 0.0529
mVec[0] = 0.5961
hVec[0] = 0.3177
nVec[1999:7999] = Ie
Ivector[
# Loop to calculate next values
for v in range(len(timeVec)-1):
= 0.01*(voltageVector[v] + 55) /
alpha_n 1-np.exp(-0.1*(voltageVector[v]+55)))
(= (0.1*(voltageVector[v]+40))/
alpha_m 1-np.exp(-0.1*(voltageVector[v]+40)))
(= 0.07*np.exp(-.05*(voltageVector[v]+65))
alpha_h = 0.125*np.exp(-.01125*(voltageVector[v]+55))
beta_n = 4*np.exp(-.05556*(voltageVector[v]+65))
beta_m = 1 / (1 + np.exp(-0.1*(voltageVector[v]+35)))
beta_h
#############
# if timeVec[v] >= 300:
# = # Part one
# if timeVec[v] > 600:
# = # Part two
##############
# Update time constants
= 1 / (alpha_n + beta_n)
tau_n = 1 / (alpha_m + beta_m)
tau_m = 1 / (alpha_h + beta_h)
tau_h
# Update gating variables
= alpha_m/(alpha_m + beta_m)
pm = alpha_n/(alpha_n+ beta_n)
pn = alpha_h/(alpha_h + beta_h)
ph +1] = pm + (mVec[v] - pm)*np.exp(-dt/tau_m)
mVec[v+1] = pn + (nVec[v] - pn)*np.exp(-dt/tau_n)
nVec[v+1] = ph + (hVec[v] - ph)*np.exp(-dt/tau_h)
hVec[v
# Update voltage
= gMaxL + gMaxK*(nVec[v]**4) + gMaxNa*(mVec[v]**3)*hVec[v]
denominator = c / denominator
tauV = ((gMaxL)*EL + gMaxK*(nVec[v]**4)*EK +
Vinf *(mVec[v]**3)*hVec[v]*ENa + Ivector[v])/ denominator
gMaxNa+1] = Vinf +
voltageVector[v-Vinf)*np.exp(-dt/tauV)
(voltageVector[v]
# Plot the results
1, figsize=(10,10))
plt.figure(4,1,1)
plt.subplot(
plt.plot(timeVec,voltageVector)'Hodgkin and Huxley Simulation')
plt.title('Voltage in mV')
plt.ylabel(4,1,2)
plt.subplot(
plt.plot(timeVec, mVec)'g_Na activation variable m')
plt.ylabel(4,1,3)
plt.subplot(
plt.plot(timeVec, hVec)'g_Na inactivation variable h')
plt.ylabel(4,1,4)
plt.subplot(
plt.plot(timeVec, nVec)'g_K activation variable') plt.ylabel(
Part 2 Unfortunately, there is no effective treatment to BTX poisoning. However, in theory, the membrane depolarization can be reversed using tetrodotoxin (TTX), which is produced by the pufferfish, blue ringed octopus, and other deadly creatures. This toxin also causes paralysis. However, unlike BTX, TTX prevents action potentials by binding to voltage-gated sodium channels and preventing the movement of sodium ions into the cell. Revise the above code to simulate exposure to TTX 300 ms after the BTX poisoning.
Part 3 Observe the behavior of the neuron between 600 and 800 ms. While this looks like more ideal behavior than when the neuron was exposed to only BTX, what should the behavior of the neuron be if neither poison had been administered? (Note, while this is a conceptual question, feel free to alter the code to see what should occur).